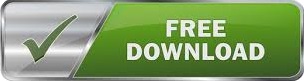
Mel-frequency cepstrum coefficients (MFCC) can be obtained by performing Cepstrum analysis on the Mel-spectrum features. Considering that human auditory perception is only concentrated on specific frequencies, methods can be designed to selectively filter the audio frequency spectrum signal to obtain Mel-spectrum features. Mel-spectrum features are commonly used in speech-related multimodal tasks, such as speech recognition. In addition, this also prompts researchers to build diverse talking face datasets and establish fair and standard evaluation metrics. The requirement poses stringent requirements on the talking face models, demanding realistic fine-grained facial control, continuous high-quality generation, and generalization ability for arbitrary sentence and identity. As highlighted in Uncanny Valley Theory , if an entity is anthropomorphic but imperfect, its non-human characteristics will become the conspicuous part that creates strangely familiar feelings of eeriness and revulsion in observers. Toward conversational human-computer interaction, talking face generation requires techniques that could generate realistic digital talking faces that make human observers feeling comfortable. Last but not least, to obtain a natural and temporally smooth face in the generated video, careful post-processing is inevitable.
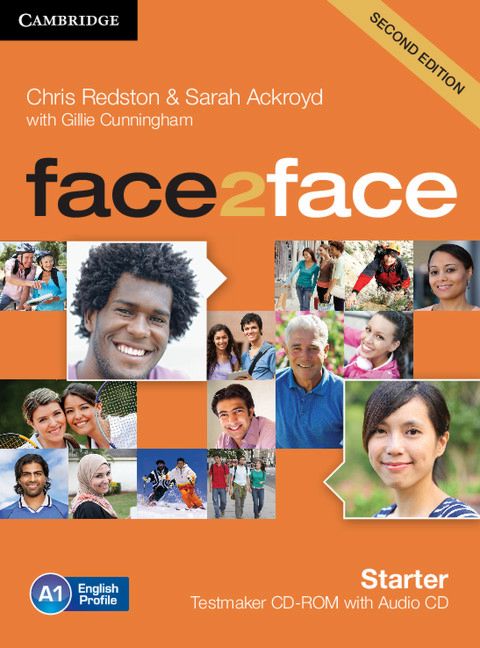
Sophisticated methods are needed to accurately and consistently match a speaker’s mouth movements and facial expressions to the source audio. As the bridge that joins audio and face, audio-to-face animation is the key component in talking face generation. Models that characterize human faces have been proposed and applied to various tasks . As the target of talking face generation, face modeling and analysis are also important.
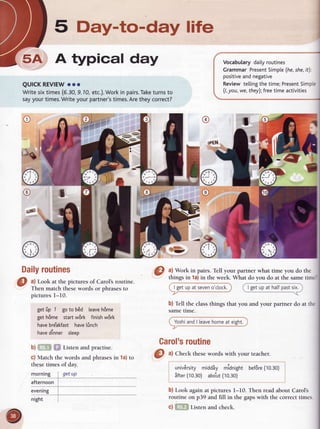
To extract essential information that is useful for talking face animation, one would require robust methods to analyze and comprehend the underlying speech signal . As the source of talking face generation, voice contains rich content and emotional information. Existing methods typically decompose the task into subproblems, including audio representation, face modeling, audio-to-face animation, and post-processing. Talking face generation is a complicated cross-modal task, which requires the modeling of complex and dynamic relationships between audio and face.

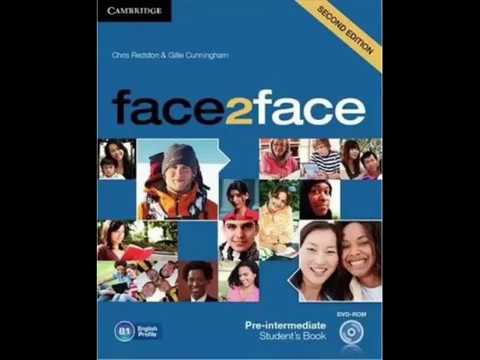
Furthermore, this technology has the potential to realize the digital twin of real person . Moreover, the animated characters enhance human perception by involving visual information, such as video conferencing , virtual announcer , virtual teacher , and virtual assistant .
#Face to face starter audio tv#
First, this technology can help multimedia content production, such as making video games and dubbing movies or TV shows . With the advancement of computer technology and the popularization of network services, new application scenarios emerged. Talking face generation has been studied since 1990s , and it was mainly used in cartoon animation or visual-speech perception experiments . Thanks to the emergence of deep learning methods for content generation , talking face generation has attracted significant research interests from both computer vision and computer graphics . Talking face generation aims at synthesizing a realistic target face, which talks in correspondence to the given audio sequences.
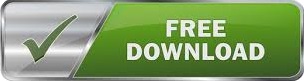